Data analytics and data science involve working with data to gain deep insights and predict outcomes. However, while there are similarities between both concepts, there are also distinct differences.
So how does data analytics vs data science compare and which approach is right for your business needs? Let’s find out.
Data science vs data analytics: A brief summary
Data analytics and data science help us better understand big data and are key elements of business intelligence. Yet differentiating between the two can be confusing. Both are interconnected, but they produce different results and pursue different goals.
While data science often involves using data to create solutions and models for predicting outcomes, data analytics is typically focused on analysing past data to support real-time decisions.
If you aim to leverage the data that your business is producing, it’s essential to comprehend what sets these two fields apart, what they offer and the value they deliver.
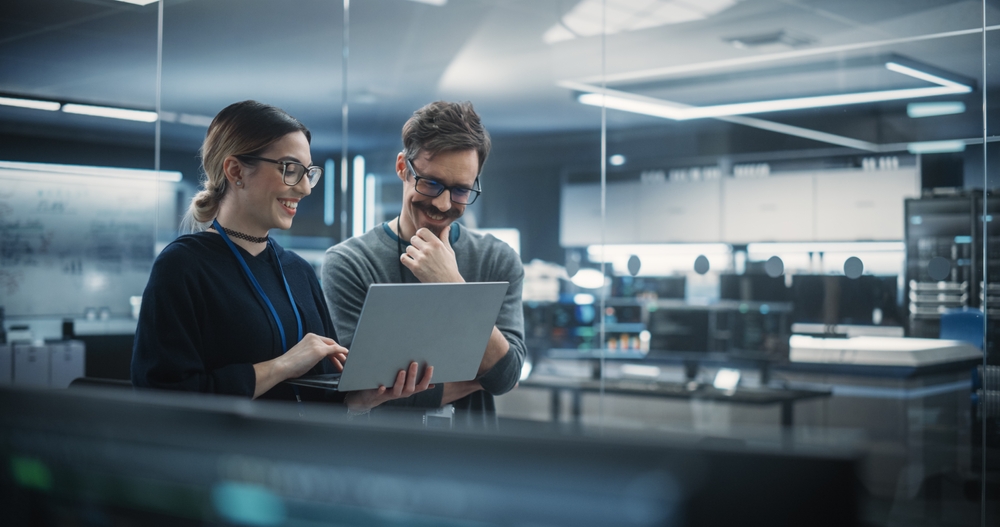
What is data science?
Data science is a broad, all-encompassing term that combines a wide range of disciplines such as mathematics, computer science and software engineering. Its chief aim is data collection and discovering patterns in large datasets to build artificial intelligence applications, develop new algorithms, train machine learning models and more.
Think of data science as a way of asking questions while digging deep into data to produce broad insights and identify areas to study. It is more concerned with asking the right kind of question than unearthing specific answers. Data scientists achieve this by training machine learning models to predict trends, create frameworks and find solutions to problems before they even rise.
Other examples of data science include:
- Collaborative filtering to produce key recommendations
- Predictive forecasting of short and long-term outcomes based on activity patterns
- Solve business problems and identify opportunities for growth
- Segmentation based on unique attributes
- Fraud detection based on anomalies and suspicious activity
- Automated tasks, processes and decision-making based on past activity and model parameters
What is data analytics?
Data analytics falls under the data science umbrella. Data scientists may leverage data analytics to gain a thorough understanding of a specific dataset or to predict potential outcomes.
Put simply, data analytics is concerned with solving problems for which we know we don’t yet have the answers and producing results that lead to measurable improvements.
Organisations often run data analytics within business intelligence (BI) platforms to improve their knowledge of current market conditions and trends. Many features of data analytics including predictions are powered by machine learning algorithms that have been developed by data scientists.
Examples of data analytics in use include:
- Actionable insights for smarter, data-driven decisions
- Identifying bottlenecks and optimising processes for improved business performance
- Customer data analysis to uncover preferences and improve products and services
- Achieve a competitive advantage by spotting market trends early
- Leverage data and insights to uncover operational efficiencies and reduce unnecessary costs and expenses
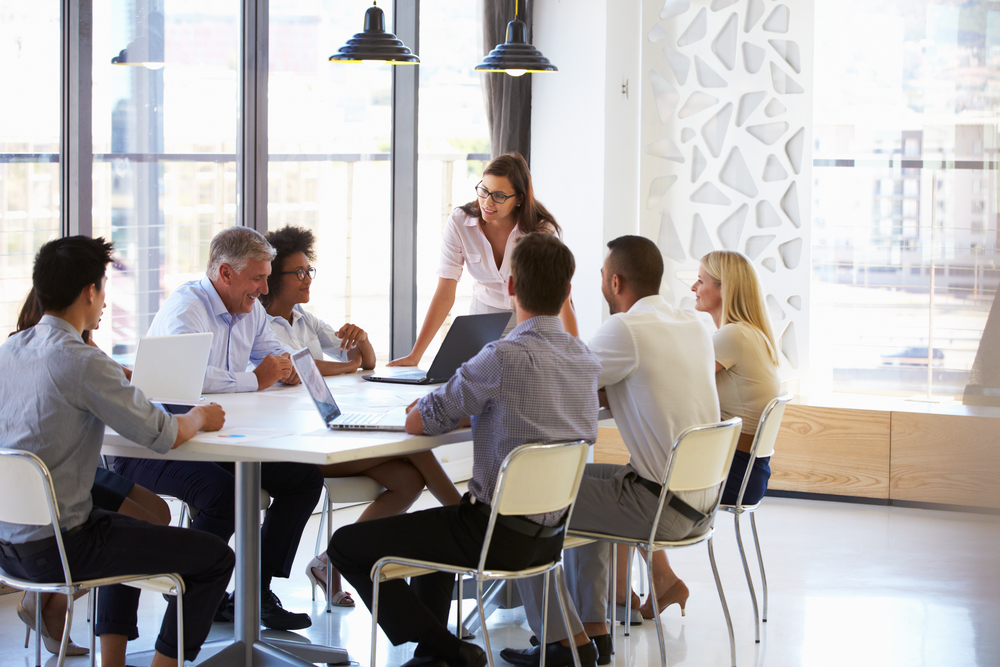
Data science vs data analytics: what’s the difference?
The concepts of ‘data science’ and ‘data analytics’ are often used interchangeably even though they are distinctly unique. The biggest difference between the two is the scope.
Data science is the overarching term for a set of fields used to mine vast datasets. On the other hand, data analytics provides a more targeted approach aimed at achieving actionable insights based on past queries that businesses can adopt to improve their operations.
Another important difference between data science and data analytics revolves around exploration. Data science is designed to break down large datasets to gain insights rather than answer specific queries. Data analytics, however, is better at using existing data to come up with answers and solutions.
With data science, you’ll achieve broader insights around key questions that should be asked. Data analytics seeks to find the answers to the key questions already being asked. Essentially, data analytics answers, ‘what happened,’ while data science explores, ‘what will happen’ and ‘why.’ Even though data science and data analytics are two different concepts, they are still unquestionably intertwined.
Here’s a summary of the key differences of data science vs data analytics:[AS1]
1. Primary purpose
Data science | Data analytics |
To produce broad insights by exploring data and answering specific queries. | To produce insights to answer specific queries in an actionable way. |
2. Type of approach
Data science | Data analytics |
To explore vast data sets for the development of models and algorithms that deliver business insights, support collaboration with stakeholders and clearly define project goals. | To prepare and analyse well-defined datasets that help identify trends and create visual aids such as graphics and charts that enable businesses to make smarter, data-driven decisions. |
3. Scope
Data science | Data analytics |
A multifaceted field that includes machine learning, statistics, data engineering and computer science. It offers predictive analytics and presents those findings to audiences in a simple and digestible way. | A wide field of analytics features which includes full data integration and deep data analysis that draws actionable insights to improve decision-making and achieve better business outcomes. |
So, can we accurately compare data science and data analytics?
Data science and data analytics are heavily connected, but they are not the same thing.
Data science is great at dissecting large datasets, providing observations, and identifying future trends. It is adept at delivering key insights that drive business success, and it can be particularly beneficial when improving AI algorithms and machine learning models. Data science asks those all-important questions that perhaps we were unaware of but stops short of giving concrete answers.
However, incorporating data analytics into the process allows us to turn all those unknown grey areas into actional insights with real-life outcomes. Therefore, it’s better to avoid direct comparisons between data science and data analysis as two separate disciplines. Instead, it is more beneficial to consider them as individual elements in a much wider process that helps us understand the information and data in our possession and how to analyse and review it for maximum benefit.
Does your organisation need help deciding between data science vs data analytics?
At Valcon, we leverage data science and data analytics to deliver sustainable business transformation across a range of industries. If you’re interested in future-proofing your organisation, contact us today.